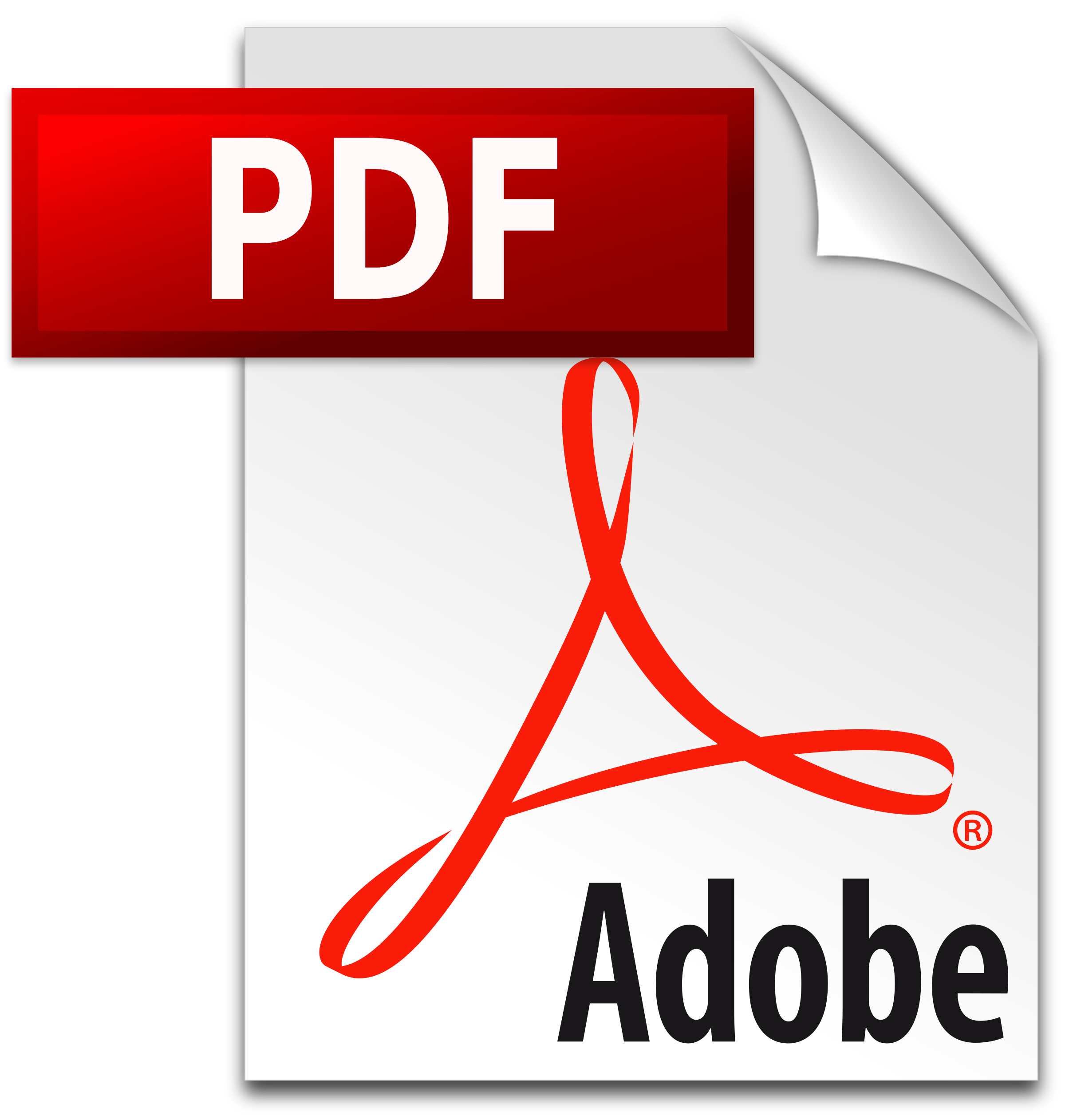
تحديد الأجهزة المكتبية ومراقبتها باستخدام تصنيف الطاقة القائم على التعلم العميق في المباني الذكية pdf
ملخص الدراسة:
Analysis of electrical energy metering profiles has experienced a substantial increase of research activity in recent years. This smart metering is a tool for monitoring energy usage and users’ behaviors as a pre-requisite for substantial energy savings. Instead of having a sensor at each appliance, non-Intrusive Load Monitoring (NILM) provides a cheaper solution by disaggregating the load data from a single meter using digital signal processing. Different algorithms have been successfully applied to a variety of load scenarios. Load data for small office appliances is available in the BLOND data set (Building-Level Office eNvironment Dataset) such as laptops, computer monitors, etc. The potential energy saving of each small appliance cannot be neglected, particularly in large companies/institutes. In this paper, a recurrent neural network (RNN) with long-short term memory (LSTM) is designed, trained, and validated for NILM on small power office equipment provided in the BLOND data set. A comparison to combinatorial optimization and factorial hidden Markov models using five metrics for performance testing shows good results for the proposed RNN.
توثيق المرجعي (APA)
El Astal, Mohammed, Abu-Hudrouss, Ammar M., Frey, Georg,& Kalloub, Mohammed (2020). Office Appliances Identification and Monitoring using Deep Leaning based Energy Disaggregation for Smart Buildings. IECON 2020 The 46th Annual Conference of the IEEE Industrial Electronics Society, IEEE. 28502
خصائص الدراسة
-
المؤلف
El Astal, Mohammed
Abu-Hudrouss, Ammar M.
Frey, Georg
Kalloub, Mohammed
-
سنة النشر
2020-10
-
الناشر:
IEEE
-
المصدر:
المستودع الرقمي للجامعة الإسلامية بغزة
-
نوع المحتوى:
Conference Paper
-
اللغة:
English
-
محكمة:
نعم
-
الدولة:
فلسطين
-
النص:
دراسة كاملة
-
نوع الملف:
pdf